Dr. Mutlu Mete
Successful classification of cocaine dependence using brain imaging: a generalizable machine learning approach
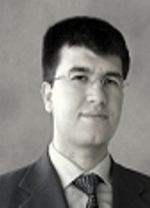
|
Speaker:
Mutlu Mete, Ph.D
Associate Professor
Date: Thu, 09.20.2018
Time: 4:30-5:30pm
Journalism Bldg-Room:129
Host: Dr. Abdullah Arslan
|
Neuroimaging studies have yielded significant advances in the understanding of neural processes relevant to the development and persistence of addiction. However, these advances have not explored extensively for diagnostic accuracy in human subjects. The aim of this study was to develop a statistical approach, using a machine learning framework, to correctly classify brain images of cocaine-dependent participants and healthy controls. In this study, a framework suitable for reducing potential brain regions that differed between the two groups was developed and implemented. Single Photon Emission Computerized Tomography (SPECT) images obtained during rest or a saline infusion in three cohorts of 2– 4-week abstinent cocaine-dependent participants (n = 93) and healthy controls (n = 69) were used to develop a classification model. An information theoretic-based feature selection algorithm was first conducted to reduce the number of voxels. A density-based clustering algorithm was then used to form spatially connected voxel clouds in three-dimensional space. A statistical classifier, Support Vectors Machine (SVM), was then used for participant classification. Statistically insignificant voxels of spatially connected brain regions were removed iteratively and classification accuracy was reported through the iterations.